Contributing to counter-racism strategies, this research analyses two data sets of survey experiments to give recommendations on how to react to subtly racial messages by the sitting President Donald Trump.The paper finds that pointing racism out works and should hence be done to make voters aware of the socially not acceptable behaviour. Furthermore, the limited impact from a single intervention on binary decisions encourages campaigners to call out repeatedly. Lastly, eroding the support for Donald does not increase the favour of his opponent. Therefore, the paper recommends accompanying the counter-racist interventions with positive advertisement on the own candidate. Otherwise, pointing out racism undermines favour for either candidate and might lead to less democratic participation.
When addressing voters with different stances on racism (from racially liberal to racially conservative), the party is confronted with the question whether calling out racism impairs Trump’s reputation or reinforces support. Both answers find theoretical backing: The theory of Racial Priming suggests that pointing out racism (especially if subtle and not immediately noticed) decreases support for the sender across all levels of racism. Theoretically, the effect increases for more racially conservative voters as they tend to face the strongest social censure. A counter-strategy based on Racial Priming thus recommends pointing out racism especially when directed at racially conservatives. In contrary, Motivated Reasoning theory argues that the exposure of (subtle) racism among racially conservatives leads to increased support for the racist speaker as the accusation of being racism is deflected to other (motivated) reasons. A Motivated Reasoning-grounded strategy calls for reluctance to point out subtle racism in front of racially conservatives. In the following, this data essay tries to identify evidence for either one of the mutually exclusive theories.
Data Sets
The data essay analyses two data sets drawn from survey experiments to construct explanatory linear and binary logistic regression models. The first data set (“Survey Data”) collected answers of 1,011 white participants that were all exposed to a faked, racist Trump TV ad and an online news article about the ad. Referring to crime, the ad accentuates crime problems with pictures of black adolescent. After being split in four treatment and one control group, the treated participants read another article in which either a white Democrat, a black Democrat, a white Republican, or a black Republican points out the racism in the Trump ad. All participants then answered a set of questions regarding their sentiment towards Donald Trump and Hillary Clinton, appreciation of non-white relatives, or their presidential vote, as well as data regarding their personal background (age, gender, income, education, etc.). In section 3, the essay uses the experiment survey data to analyse the treatment effect on different levels of racial liberalism/conservatism with regards to favour for Trump and Clinton, the difference between both, the likelihood to vote for Trump, and the declaration of the ad being racist. Furthermore, the essay looks at possibly different effects of participants of the treatment group being appealed by a white Democrat, a black Democrat, a white Republican, or a black Republican.
The second data set (Tweet data) intends to replicate the first data set with 595 white participants, recruited via Amazon’s Mechanical Turk, a crowdsourcing Internet marketplace that coordinates microtasks between firms and independent workers. In this experiment design, the treatment group was provided a news article referring to a Trump tweet that the article calls out racist. The control group received an article about a mobile phone and was neither informed about the tweet nor the indication of racism. Afterwards, control group and treatment group answered a comparable but smaller set of questions as in the Survey Data experiment. Trying to replicate the finding of the first analysis, the essay first analysis the treatment effect in this setting and then set ups three binary logistic regressions assessing the approval of Donald Trump handling his job as President, his handling of crime, and the declaration of racism in his tweet.
Both datasets were analysed using the OpenSource Software R and R Studio interface. The R code can be found in the Appendix. The essay uses the libraries “foreign”, “texreg”, “lmtest”, “sandwich”, “stargazer”, and “tidyr”. The significance level throughout the whole analysis is α = 0.05.
Survey Data Analysis
Drawing on the previous research, racially conservatives show more support for Donald Trump than racially liberal. Against this backdrop, the essay first checks for a significant difference in racial conservatives being appealed by different politicians. Finding no difference, the paper then demonstrates the influence of treatment on the favour for Trump, Clinton, the difference between Clinton and Trump, the voting behaviour and the declaration of the ad being racist – finding evidence for the Racial Priming theory.
Difference in the appeal by white or black, Democratic or Republican politician
Arguing theoretically, one could expect white racially conservatives to respond differently to appeals by different politicians. Reading about “one of their kind”, i.e., a white Republican, pointing out and excoriating racism could have a stronger effect than if heard from a black Democrat. To detect a significant difference between altering persons of appeal, the paper conducts t-tests for difference in means between the different treated groups. The t-test follows the null and alternative hypothesis:
- H0: There is no difference in the level of declaring the ad as racist when a racially conservative is being appealed by a white Republican (Paul Ryan), a black Republican (Ben Carson), a white Democrat (Bernie Sanders) or a black Democrat (Barack Obama).
- H1: There is a significant difference in the level of declaring the ad as racist when a racially conservative is being appealed by a white Republican, a black Republican, a white Democrat or a black Democrat.
To define the group of racially conservatives, the paper divides the treated participants at their median of racism level (variable “raceresent”). “Raceresent” is continuously scaled, ranges from -3.7 (most racially liberal) to 2.55 (most racially conservative) and the median for all treated participants is at 0.25. The median is preferred over the mean as it divides racial liberals and racial conservatives in two equally large groups (number of treated participants) and does not balance out the groups with regards to their degree in racism. Thus, extreme values have less influence – an approach usually referred to in political science.
The essay conducts t-tests for difference in means for three most interesting variables, finding no difference by whom the appeal is made. This finding helps to reduce complexity in the following regression models: There is no need to differentiate between persons of treatment.
Five Regression models to detect treatment effect
The overarching goal of all regression models is to determine whether the experiment data supports the Theory of Racial Priming, Motivated Reasoning Theory, or none of them and whether it is
advisable to counter racist messages. As the influence of the treatment lies at the centre of this analysis, the models use only “treat2”, “raceresent”, and an interaction as independent
variables to avoid polluting results. The paper refines the counter-strategy with insights from the second data set.
Table 1 summarizes the final models to regress the treatment effect on candidate evaluation, voting for Trump, and thinking ad is about race:
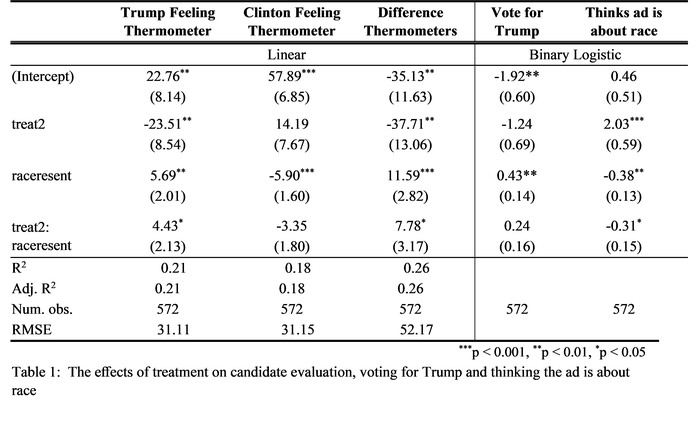
Model 1: Trump Feeling Thermometer
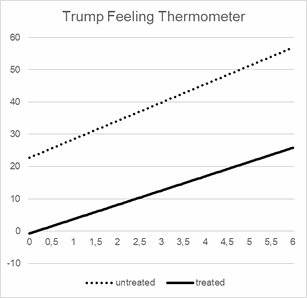
In line with previous research, the model regression on Trump Feeling Thermometer shows an increase in Trump favour for increasing levels of racial conservatism. Per one unit increase in the level of racial conservatism, the favour for Trump raise by 5.69 percentage points. The treatment shifts the overall level of favour from 22.76 percent favour (intercept) by -23.51 percentage points down, reducing s favour for Trump. The interaction points out that with increasing level of racial conservatism the increase in favour grows slower (β-coefficient: 4.43). Figure 2 illustrates the findings. The interpretation in this model is clear: Pointing out racism reduces the overall favour of Trump and effects particularly strong those who are more racially conservatives. The findings support the Racial Priming Theory.
Model 2: Clinton Feeling Thermometer
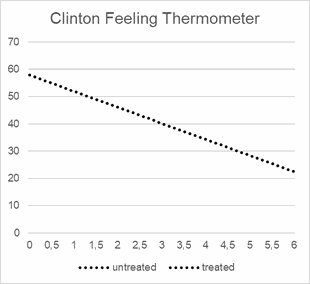
Opposite to the TRT, favour of Clinton among racially liberal is high (57.89) and decreases the more racist participants are (β-coefficient: -5.90). As neither treatment nor interaction are significant, being exposed to the ad, the article and the pointing out of racism does not affect the favour for Clinton. This seems theoretically plausible as the reduction of favour for one candidate does not necessarily raise the favour for the other (see Figure 3).
Model 3: Difference in Feeling Thermometers
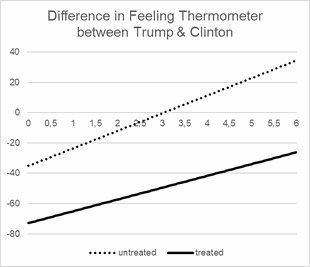
Drawing from the previous two regression, the results here are no surprise but back the presented finding: As with an increase in racial conservatism favour for Trump rises and favour for Clinton decreases, it is little surprising to see a strongly upward leaning slope for “raceresent” (β-coefficient: 11.59). Significant treatment shifts the overall graph by -37.71 percentage points towards Clinton and reduces the strong increase with higher levels of racial conservatism to a slope of 7.78. In line with insights from the first model, the finding strongly favours the Theory of Racial Priming as racially more conservative shift their favour from Trump to Clinton more severely (see Figure 4).
Model 4: Vote for Trump
In line with previous research, the level of racial conservatism increases the likelihood to vote for Trump. Apart from their algebraic sign, β-coefficients of Logit models (indicating a change in log-odds ratios) are difficult to interpret. Adjusting them to odds ratios signals that an increase of one unit on the “raceresent” scale increases the likelihood to vote for Donald Trump by 54 %, holding treatment level constant. As the variables are insignificant, the treatment neither effects the overall level of voting for Trump, nor does it affect the change in likelihood with increasing levels of racial conservatism. This finding contrasts the linear model 1-3 which indicate a strong reduction of increase in favour for Trump after being exposed to the article pointing out racism. A possible interpretation for the differing results: The influence of one negative case of Trumps behaviour might not be influential enough to initiate a change in voting behaviour. Meaning, the appreciation of Trump might have lowered but is still sufficiently high to outweigh Clinton. Furthermore, one single intervention seems insufficient to affect long standing political convictions.
Model 5: Thinking Ad is about Race
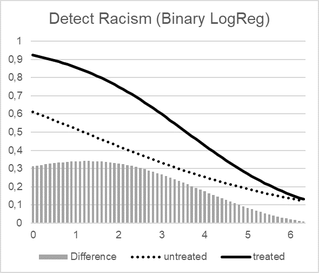
With regards to the results, increasing level of racial conservatism lead to less likely declaration of racism. A one unit increase in racism reduce the odd ratios of declaring the add as racists by 54 % (assuming that treatment is controlled for). The effect could be theoretically explained by less sensitivity for subtle racism at higher levels of racial conservatism – an explanation neither attributable to the Racial Priming, nor Motivated Reasoning as both theories seek to explain behaviour after being confronted, not beforehand. Being racist can stem from old societal pattern, adopted by individuals unknowingly and thus being less sensitive to the issue. These “ignorant racists” are less likely to point out racism as they do not identify it as such.
Regarding the treatment effect, the coefficients indicate a significant shift towards a higher likelihood of declaring the add as racist. Being treated increases the odds of declaring the ad as racism by 661 %. This seems obvious as it’s been pointed out in the article the participants just read. The interaction term follows the trend of “raceresent”, where higher levels of racial conservatism lead to a less likely declaration of racism; yet, the treated effect for higher levels of racism is not as strong as the untreated effect (untreated: reduction through multiplication with 54 %; treated: reduction through multiplication with 64 %). Interestingly, the difference between treated and untreated log-odds ratios converges for higher level of racism. This stands in contrast to the previous finds of the linear regressions where higher level of racial conservatism always led to stronger effects when treated (in comparison to untreated). A rather bold interpretation might solve the discrepancy by arguing that extreme racial conservatives are strongly affected by social census and hence adjust their behaviour accordingly (Racial Priming Theory). But when explicitly asked to point out racism, they avoid doing so, to not experience any further social sanction. It might be more convenient to deny the existence of racism when ask, than to skitter into more social constraints.
Tweet Data analysis
As explained previously, the Tweet data set contains experimental survey data of 595 white participants, recruited via Amazon Turk and divided into a treatment and a control group. The treated read an article pointing out racism in a previous Trump tweet, the control group read an article about a mobile phone. With this data set, the paper seeks to reproduce the treatment effect of section 3 in relation to the level of racial conservatism. It therefore models three binary logistic regressions on the approval of the job done by Donald Trump, the approval of Trump handling crime, and the declaration of racism in the Trump tweet.
Reproducing the treatment effect: “jobapprv” and “crmapprv” models
The models “regJA.1.wABT” and “regHC.1.wABT” are two binary logistic regressions with the dependent variables “jobapprv” and “crmapprv” respectively. The independent variables are in both cases “raceresent” (as in previous data set), “treat” (binary variable: 0 = control group, 1 = treatment group), and the interaction of “raceresent” and “treat”. After mandatorily adjusting standard errors, “raceresent” is significant for both models, the other variables are not. The increasing approval of Donald Trump’s job and his handling of crime at higher levels of racial conservatism stand in line with the finding of the first models as well as previous research. Surprisingly, this data set shows no treatment effect – an observation that stands in contrast to the first three linear regression model yet fits the findings of the VFT logit model. The paper refers to possible reasons for this discrepancy in the discussion of this section.
Refining the treatment group via three logit models
Referring to the additional variables in the data set, age, gender, education, income, religion, and ideology seem most suitable to generate addition insights into the characteristics of treated participants approving or disapproving Trump’s job or his handling of crime, as well as the detection of racism in the tweet. It is important to highlight that this refinement is not made to show how the treatment affects different subgroups of participants but to further immerse into the specifics of the treated groups, who, for example, still show the highest support for Trump even when treated. The research is conducted to specify the target group for a counterstrategy on racism more precisely.
The additional variables are – apart from age – all ordinal or nominal scaled, requiring the transfer into dummy variables to be integrated in the logit model. All standard errors are adjusted for heteroskedasticity as required for logit models. Table 2 summarizes the final binary logistic regression models built from the Tweet data set:
Job approval – logit model
Expanding the previous “regJA.1.wABT” with the dummy variables shows that neither income, gender, nor education have a significant effect on the job approval. In line with previous research, high level of ideology, i.e., identification with the Republican party, leads to significantly higher job approval in comparison to more Democrat identification. As this finding is obvious, it is left out in the final model. More interestingly, the interaction of age with the level of racial conservatism is significant: The older and more racially conservative, the more likely to approve Trump’s doing (a one unit increase in “age” or “raceresent” leads to 3 % increase in the odds to approve Trump’s doing). Most interestingly, the religion dummy for Jewish, Atheist, and other religious groups is significant[1]. The religion dummies all point in a negative direction, indicating that being Jewish, Atheist, or belonging to a miscellaneous religious group reduces the likelihood of approving Donald Trump when compared to being Protestant. These finding are congruent with previous research, stressing a substantive electoral base for Trump among Christian voters.
Crime Handling – logit model
Like the “regJA” model, the paper also tested all dummy variables on the approval of Trump handling crime (“crmapprv”). In contrast to the job approval model, the interaction of age and raceresent is insignificant. In return, the significant effect of “raceresent” increases crime handling approval for higher level of racial conservatism – much in line with previous finding and research. The effect on the approval of handling crime is also significant and negative for all religion dummies, pointing out that in comparison to protestant (who are among the most conservative religious groups in the country) all religious groups approve less of Trump handling crime.
Declaration of Tweet as Racist
The third logit model regresses all suitable variables on the declaration of the tweet as racist. After adjusting standard errors, “raceresent”, “age”, and being Jewish turns out significant. Whereas the first variable only supports our previous finding, age is of interest: The older participants are, the more likely they declare the tweet as being racist (being one year older increases the odds of declaring racism by 2 %). A possible interpretation refers to becoming more confident about one’s opinion over time and hence daring to point out racism. Lastly, the Jew dummy is significant and positive, increasing the odds of pointing out racism by 515 %, in comparison to being Protestant (baseline). This significant effect of being Jews could be linked to higher sensitivity for Racism when belonging to a religious minority.
Discussion of model results
Summing up the finding of the Tweet data analysis, the missing evidence for a treatment effect is striking. In the following, the paper gives three possible explanations for the difference:
First, comparable to the “Vote for Trump” logit model, the single treatment with an article pointing out racism in Trump’s tweet, might not be strong enough to shift the binary decision of participants to approve or disapprove his doing. Even though the answers on job approval and crime handling approval seem not as fundamental as casting a (theoretical) vote, binary variables provide less information on the internal shift of sentiment among participants. A more refined measure of the effect or repeated treatment could help to detect the treatment effect.
Second, the data sets differ. Apart from Tweet data stemming a smaller experiment, the selection of participants was biased towards Amazon’s Mechanical Turk volunteers. Partaking in an internet-driven platform like Mechanical Turk supports the argumentation that those participants are more exposed to Trump tweets and therefore react less to a treatment. A patient used to painkillers reacts less to another pill than someone completely unfamiliarised with analgesics.
Third, participants treated in the Survey data experiment read an article and watched a TV ad, something that could be perceived as more lasting than one of Donald Trump frequent tweets. Thus, the effect of being exposed to the article and the ad could be stronger than the effect of reading a tweet, resulting in smaller chances to observe the effect in the Tweet data set.
Conclusion and Policy Recommendations
This data essay analysed two data sets seeking advice on how to counter the (subtle) racism of the sitting President Donald Trump. Regarding theoretical stances, pointing racism out publicly can have two adverse effects: Following the Theory of Racial Priming, claiming a message to be racist reduces support for a racist sender, especially among more racially conservative supporters. In contrary, Motivated Reasoning Theory argues that pointing out subtle racism leads to avoidance among racially conservatives and even increases their support for the sender.
Summary of results
Throughout both data sets, the clear link between higher racial conservatism and increased favour, support, and vote for Donald Trump is uncontested. These insights are also backed by previous research. Nevertheless, the treatment of survey participants with articles pointing out racism led to observable and significant changes in the level of favour for Donald Trump, indicating the effects of Racial Priming rather than Motivated Reasoning. Here, it does not matter who points out the racism as the first t-tests disclose. As the models on Trump feeling thermometer and the difference in thermometers indicate, the increase in favour of Trump (overall and relative) increases slower if participants were shown the article.
On the other side (and against the Democratic Party’s interest), the treatment did not affect the favour for Hillary Clinton. Higher levels of racial conservatism show less favour, the treatment is insignificant. The results underline that impairing a rival’s image does not improve the own appreciation.
Furthermore, the treatment influenced the likelihood of declaring the ad as racist, as demonstrated in the “Declaration of Racism” model based on the Survey data set. In contrast to increasing effects for more racial conservatism, the treatment does not affect the likelihood to declare racism for the very racially conservatives.
Next, the logit model “Vote for Trump” based on the Survey data set and the first logit models “Job Approval” and “Crime Handling Approval” based on the Tweet data set did not reproduce a significant treatment effect. Possible reasons for this deviation between linear and binary findings and the different data sets have been stated throughout the essay: (1) The effect of treatment is too small and one intervention not sufficient to shift a strong binary decision like voting. (2) The increasing size of the effect on racially conservatives is partially offset as the margins of favour for Trump over Clinton are also larger, hence reducing a binary shift. (3) Internet-accustomed Tweet data participants might be more used to (racist) Trump tweets as they were recruited on Amazon’s Mechanical Turk platform. (4) Survey data participants were treated with an article and a TV ad, which deems more influential, than an article about one of hundreds Trump tweets.
Lastly, the three logit models that focussed only on treated participants and were based on the Tweet data set allowed for limited insights into the characteristics of the treatment group: Being neither Protestant nor Catholic reduces the odds of approving how Trump handles his job or crime. Aging increases the likelihood of approving Trump as well as declaring racism. Beyond these insights, it is difficult to attributed clear characteristics to the supportive base of Donald Trump from this data set. The very interesting variables gender, income, and education all turned out insignificant.
Limitations
Working with experimental data in general as well as these two data sets are limited in two ways: First, the sampling of participants is, especially in the Tweet set, biased towards Amazon’s Mechanical Turk participants who deem very internet and technology interested. Second, the experimental setting allows for high internal validity, as random attribution into treatment and control group interpret the observed treatment as actual causal claim. Nevertheless, setting has limited external validity so that generalization is hampered: The experiment sample was not drawn randomly from the population as it included, for example, only white participants or very few Muslims. To advance the study of this paper, it is advisable to expand the research in an observational setting.
Policy Recommendations
Awaiting a heated election with an incumbent President Donald Trump, the question of how to counter subtle and open racism from Trump becomes crucial. The findings of this paper support several policy recommendations: (1) Pointing racism out works and should hence be done to make voters aware of the socially not acceptable behaviour. The paper recommends this strong stance against the controversial results of the binary models, as the continuous scale of the linear models provides a more information and higher sensitivity for the treatment effect and is thus preferred. (2) Due to the particularly strong impact on racially conservatives, the paper recommends targeting responses directly at them. This focus allows a double effect: The response works as a social sanction for those who are deliberately racist (in the original sense of the RP theory), and as an enlightenment for those who are “ignorant racists”, i.e., socialized with low sensitivity on the topic. (3) The limited impact from a single intervention on binary decisions, like a vote, encourages campaigners to use the call out as repetitive action to undermine the support the opponent. (4) As eroding the support for Donald does not increase the favour of his opponent, the paper recommends accompanying the counter-racist interventions with positive advertisement on the own candidate. Otherwise, pointing out racism undermines favour for either candidate and might lead to less democratic participation.
This research was submitted as final paper for the course "Statistic I" at Hertie School of
Governance, lectured by Philipp Broniecki. Please note that the research is built on limited statistical means for learning purpose . Conclusions drawn from this analysis may require further
refinement!
Write a comment